Visit Our websites
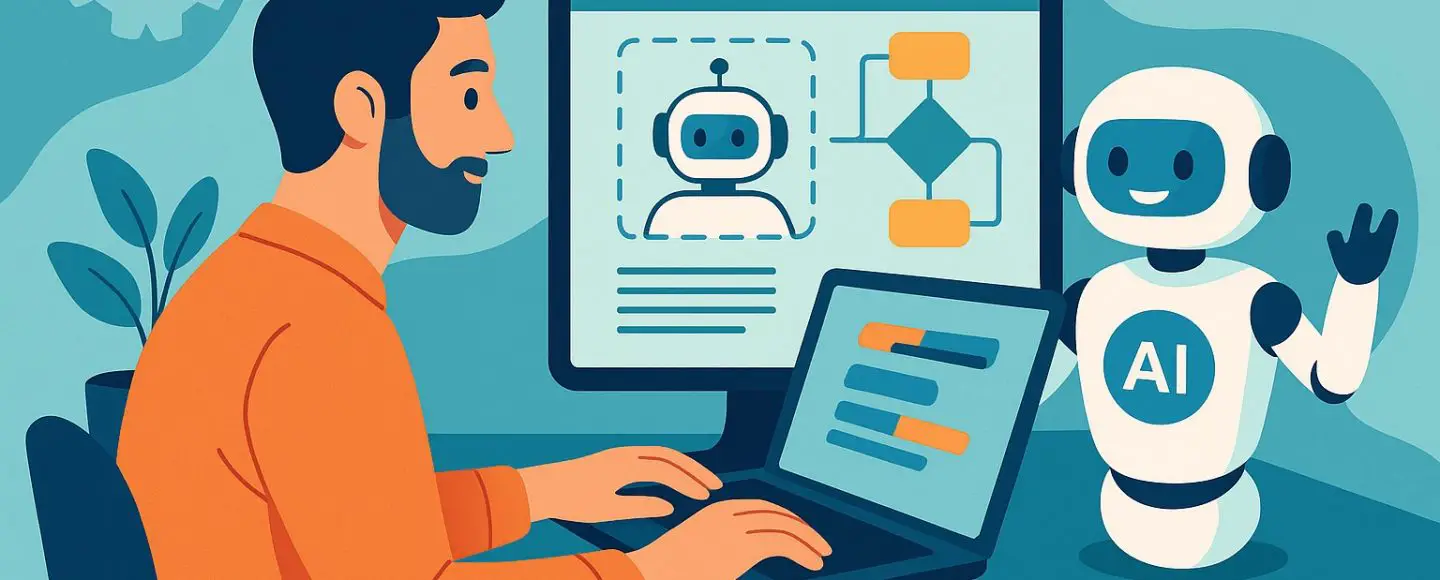
Build Custom AI Agents for Workflow Automation
Build Custom AI Agents for Workflow Automation to eliminate repetitive tasks, improve operational efficiency, and scale business operations without exponentially increasing costs. If you’re an entrepreneur, business leader, or IT professional, you know how time-consuming everyday workflows can drain resources and slow growth. Imagine transforming static tasks into dynamic, autonomous processes that work around the clock. In this guide, you’ll learn how to create your own team of AI agents, each with specialized roles, to enhance productivity and redefine how your organization operates.
Also Read: Understanding AI Agents: The Future of AI Tools
Understanding AI Agents: What They Are and Why They Matter
AI agents are designed to perform human-like tasks with little-to-no supervision. These agents operate on predefined instructions and are trained to handle specific responsibilities, from managing calendar invites, answering customer emails, generating reports, or automating sales outreach. Each AI agent can be trained on a unique dataset or workflow, which allows it to execute complex tasks in real-time.
Unlike single-purpose automation tools that often require manual triggers, AI agents use contextual awareness and can interact with other systems. This allows not just seamless automation, but also enhanced decision-making. In short, AI agents aren’t just replacing repetitive tasks—they are improving how those tasks are performed.
Traditional tools such as scripts, macros, or robotic process automation (RPA) rely on rigid rule-based systems. They can complete structured tasks but often fail when the process requires adaptability, interpretation, or problem-solving.
AI agents, on the other hand, utilize natural language processing (NLP), machine learning, and large language models (LLMs) to understand context. This allows them to make decisions, interact with users more naturally, and evolve from feedback. As a result, your AI-powered workflows can adapt over time and remain effective even as your business processes evolve.
Also Read: Unlocking ChatGPT’s Hidden Gem: Custom GPTs
Key Steps to Build Custom AI Agents for Workflow Automation
Creating AI agents for your business doesn’t require deep coding expertise anymore. With platforms like AIagent, you can build and deploy powerful agents with simple prompts. Here’s a simplified step-by-step approach:
1. Define the Workflow Objective
Start by identifying the workflow or task you’d like to automate. It could be handling website inquiries, preparing financial reports, automating helpdesk ticket resolution, or performing sales outreach.
Make sure the objective is clear and measurable. This clarity serves as the foundation for training your AI agent and aligning its behavior with desired outcomes.
2. Choose Your AI Agent Roles
Rather than building a single monolithic agent, consider assigning roles. Create specialized agents for tasks such as scheduling, data entry, documentation, and customer interaction. Dividing responsibilities makes each agent easier to manage and improves overall performance.
Examples of commonly used agents include:
- Executive Assistant Agent: Manages virtual meetings, schedules, and follow-ups.
- Customer Support Agent: Handles incoming queries from email or chat with contextual replies.
- Content Generator Agent: Writes blogs, newsletters, and product descriptions based on brand tone.
3. Train Each Agent with Contextual Knowledge
The power of a custom AI agent lies in its training data. Tools like the AIagent platform allow you to upload documents, URL links, and prior communication records that give the agent domain-specific knowledge.
For example, a support agent trained on your knowledge base and onboarding manuals can effectively resolve customer issues without human intervention. Chat-based memory and contextual lookup allow the agent to refer to multiple documents for a single task.
4. Test and Refine Agent Behavior
Once trained, begin testing the agent in a controlled environment. Give it sandbox access to test workflows before going live. Observe how it handles variation or ambiguous inputs. Make adjustments as needed by revising the input training data or refining prompt templates.
Evaluate the AI agent using defined performance metrics like accuracy, resolution time, user satisfaction scores, or reduction in human intervention. Make improvements iteratively to achieve ideal results.
5. Deploy and Integrate with Workflow Tools
Deploy the AI agents across existing platforms and systems. Connect it with APIs from tools like Slack, Trello, CRM systems (like Salesforce or HubSpot), or cloud storage providers like Google Drive or Dropbox. These integrations ensure agents work within your organizational ecosystem smoothly.
Some platforms also allow multi-agent collaboration, where each AI agent communicates with others to complete a task together. For example, a sales agent can request product sheets from a document agent before sending proposals to prospects.
Real-World Examples of AI Agent-Driven Automation
Many organizations are already using agent-based automation across different sectors. Here are a few impactful use cases:
- Startups: Deploy customer support agents that answer questions 24/7, increasing conversion rates and freeing up skilled staff for growth-related tasks.
- Human Resources: Use onboarding agents to teach new employees policies, benefits, and company culture without manual effort.
- E-Commerce: AI-powered content agents automatically write product descriptions based on inventory data.
- Healthcare: Create compliance-focused administrative assistants that manage workflows in HIPAA-compliant environments.
As these examples show, AI agents don’t replace staff—they act as intelligent extensions of human effort.
Also Read: Three Essential Benefits of AI Agents
Today’s no-code and low-code platforms make building AI agents more accessible than ever before. Common tools include:
- AIagent: A powerful platform that lets you build, configure, and deploy teams of AI agents with ease. Ideal for SMBs and creators looking for plug-and-play solutions.
- LangChain: A language modeling framework that supports advanced agent workflows and is developer-friendly.
- ChatGPT with GPT-4: Useful for experimenting with prompt engineering and building prototype agents.
Selecting the platform depends on your technical proficiency and automation goals. No-code tools are better for quick deployment, while code-based platforms offer customization for enterprise-grade applications.
SEO Considerations for AI Agents in Business Automation
When integrating AI agents on customer-facing platforms like websites and chatbots, ensure SEO alignment:
- Train AI agents to use SEO-optimized language that aligns with your brand voice.
- Use schema markup for automated content generated by website agents.
- Ensure your agents respond using keyword-rich answers to improve on-page SEO.
- Automatically update blogs or FAQs using AI content agents to keep your site fresh and relevant.
AI agents can become a core part of your content strategy, helping your business rank higher while reducing the need for manual updates.
Building a Sustainable Future with Workflow Automation
As businesses face increasing competition, rising operational costs, and growing customer expectations, intelligent automation offers a clear path forward. Implementing a team of custom AI agents ensures your workflows are agile, accurate, and scalable.
By leveraging AI to handle administrative, operational, and creative responsibilities, you shift valuable human attention to strategic decision-making and innovation. Building an AI agent workforce is not just about reducing workload—it’s about enabling growth and transformation.
Whether you’re a solo entrepreneur, small business, or enterprise leader, now is the time to embrace AI agent automation. The tools are ready, the process is proven, and the competitive edge is undeniable.
Also Read: AI Agents in 2025: A Guide for Leaders
References
Anderson, C. A., & Dill, K. E. The Social Impact of Video Games. MIT Press, 2021.
Rose, D. H., & Dalton, B. Universal Design for Learning: Theory and Practice. CAST Professional Publishing, 2022.
Selwyn, N. Education and Technology: Key Issues and Debates.Bloomsbury Academic, 2023.
Luckin, R. Machine Learning and Human Intelligence: The Future of Education for the 21st Century. Routledge, 2023.
Siemens, G., & Long, P. Emerging Technologies in Distance Education. Athabasca University Press, 2021.
BONUS LINK VISIT NOW
Visit Our websites